重い不用品は業者に依頼するのがおすすめ
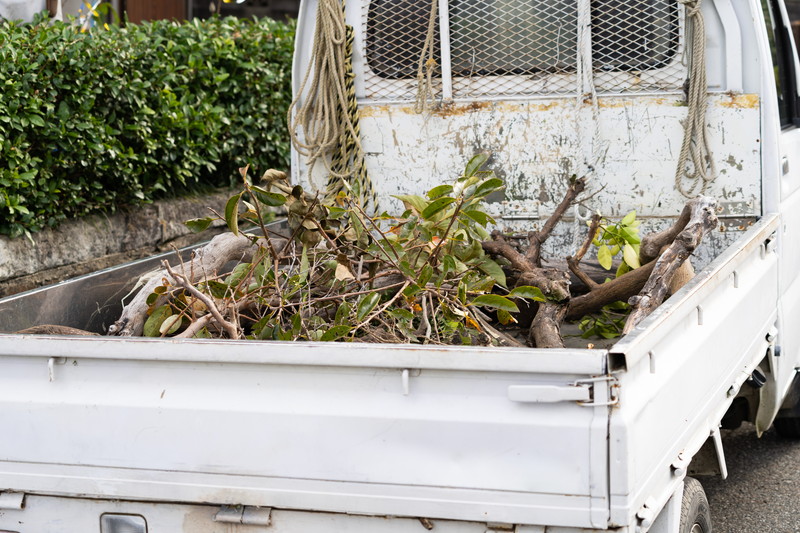
家具や家電製品など重い不用品は、業者に依頼するのが最もよい方法です。
まず、自分で不用品を移動させなくて済みます。重い不用品を処分するときには、住んでいる自治体の粗大ごみ回収を利用するか、粗大ごみを自治体のごみ処理施設に持ち込むか、不用品回収業者や遺品回収業者を利用するのが一般的です。しかし、自治体の粗大ごみを利用する場合と自治体のごみ処理施設に持ち込む場合は、回収場所まで自分で不用品を運ばなければなりません。無理をして運ぼうとするとケガにもつながりかねないため、重いものを運ぶのに慣れていない方には危険な方法です。
次に、粗大ごみの回収が行われたり自治体のごみ処理施設が開いたりしているのは、平日の日中であるところがほとんどです。業者の中には平日だけでなく、休日や早朝・夜間も営業しているところがあるため、忙しい人も依頼しやすいです。
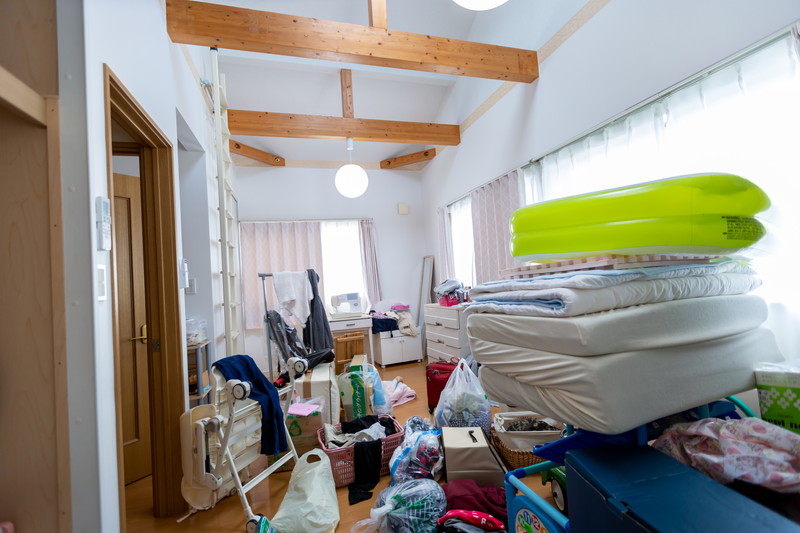
それに、自治体の粗大ごみの回収は、自治体に電話をしてから回収日まで時間がかかる場合もあります。ほかにも一度に回収してもらえる点数が決められている自治体が多いですし、利用者を住民に限定している自治体もあります。空き家の整理や遺品の片付けなどで、その家の住民でない方が片付けをするときに利用できないケースもあります。業者であれば、その場所に住んでいない方も依頼できますし、中には当日の立ち会いがいらない業者もあります。
中にはパソコンやテレビ、エアコン、冷蔵庫など、自治体の粗大ごみでは回収してくれないごみもあります。一方、業者なら自治体の粗大ごみでは回収していないごみも処分してくれるのが一般的です。分別できないごみも、業者のスタッフがやってくれるので安心です。
業者の中には悪徳な業者もあるのは確かです。ただ、「一般廃棄物処理業」の許可を受けた業者で、業者のスタッフの顔写真がホームページに掲載されている業者は良心的なところが多いです。ホームページに寄せられた顧客の口コミなども参考に、業者を選ぶとよいでしょう。